Özyeğin University, Çekmeköy Campus Nişantepe District, Orman Street, 34794 Çekmeköy - İSTANBUL
Phone : +90 (216) 564 90 00
Fax : +90 (216) 564 99 99
E-mail: info@ozyegin.edu.tr
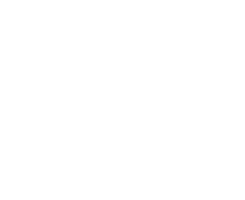
Thesis Defence – Efe Mert Ustaoğlu (MSFE)
Efe Mert Ustaoğlu- M.Sc. Financial Engineering
Asst. Prof. Dr. Emrah Ahi – Advisor
Date: 13.05.2025
Time: 13:30
Location: Özyeğin University Altunizade Campus - Classroom ALT 101
“Forecasting The Turkish Yield Curve Using Deep Learning And Econometric Models: A Comparative Analysis”
Asst. Prof. Dr. Emrah Ahi, Özyeğin University
Asst. Prof. Dr. Levent Güntay, Özyeğin University
Asst. Prof. Dr. Rıza Ergün Arsal, İstanbul Bilgi University
Abstract:
This thesis investigates the modeling and forecasting of the Turkish Treasury bond yield curve using both traditional time series models and deep learning approaches. The study focuses on evaluating the performance of models such as Autoregressive (AR), Random Walk (RW), the Dynamic Nelson-Siegel (DLNS) model with fixed and time-varying decay parameters, and Long Short-Term Memory (LSTM) networks. Daily zero-coupon yield data between July 2012 and February 2025 were used, covering maturities ranging from 3 months to 10 years. Forecasting performance was assessed using an out-of-sample expanding window framework for different horizons. Root Mean Squared Error (RMSE) was employed as the main accuracy metric, and the Diebold-Mariano (DM) test was applied to determine whether performance differences across models were statistically significant. The empirical findings show that while simpler models such as AR and RW perform well in the short term, their forecasting accuracy deteriorates as the horizon increases. In contrast, LSTM models—particularly those trained on latent factors derived from the Nelson-Siegel framework—demonstrate superior generalization performance in medium- and long-term forecasts. Notably, the DLNS model with a time-varying λ also outperforms benchmark models at longer horizons. These results highlight the value of incorporating structured factor dynamics and deep learning techniques for forecasting complex financial time series such as the yield curve.
Keywords: Yield Curve, Deep Learning, Nelson-Siegel Model, LSTM, Time Series Forecasting, Diebold-Mariano Test, Bond Yields, Turkish Treasury Bonds
Bio:
Efe Mert Ustaoğlu holds a bachelor’s degree in econometrics and currently works at the Banking and Financial Institutions Department of the Central Bank of the Republic of Turkey. He is doing a master’s degree in the FERM program, focusing on yield curve forecasting using deep learning techniques.